Imagine wanting to build a house.
β
What do you need first? A design.
β
Who do you turn to? The expert - an architect.
β
In business, there is also a counterpart for this: a data engineer.
β
The data engineer is like the architect who designs and plans the structure. They ensure that the foundation is strong, the plumbing and electrical systems are in place, and the framework is ready. They create a solid infrastructure for the house to stand on.
β
After the blueprint of the house, it would also be ideal to have a design for the inside of your home.
β
This is where interior designers are experts.
β
Interior designers take the finished house and turn it into a comfortable and functional living space. They choose the furniture, colors, and dΓ©cor that make the house welcoming and functional for its inhabitants' needs.
β
In business, it's the data scientists who are the interior designers.
β
Data scientists analyze and interpret the data that data engineers have prepared. They then turn these into actionable insights and valuable information for any business.
β
β
In the digital age, data has transformed into a priceless asset for businesses across various industries.
β
With the widespread use of technology and advanced data collection methods, organizations now have access to large volumes of data.
β
This accumulated data contains valuable insights that can shape and drive decision-making processes. This can lead to improved efficiency, enhanced customer experiences, and a competitive edge in the market.
β
Data Engineers and Data Scientists are leading the way in this data-driven revolution.
β
Though both deal with data, Data Engineers and Data Scientists have distinct roles and functions. This makes them vital in their respective fields.
β
In this blog, we will explore the unique worlds of Data Engineers and Data Scientists and gain insights into their specialized domains.
β
We specifically discuss:
- What Data Engineers do
- Why Data Engineering is Important
- What Data Scientists do
- Why Data Science is Important
- Compare Data Engineers and Data Scientists
- The future demand for Data Engineers and Data Scientists
β
What do Data Engineers do?
Data engineers are the architects behind the scenes, designing, building, and maintaining the intricate systems that facilitate the movement of data.
β
Their competence ensures that data is readily available, secure, and well-organized, empowering organizations to make research-backed decisions and derive valuable insights to stay ahead in today's competitive landscape.
β
Data Engineers are the masterminds behind the creation of data systems that serve as the backbone of modern organizations' data operations.
β
The key tasks of Data Engineers include:
β
1. Data Infrastructure
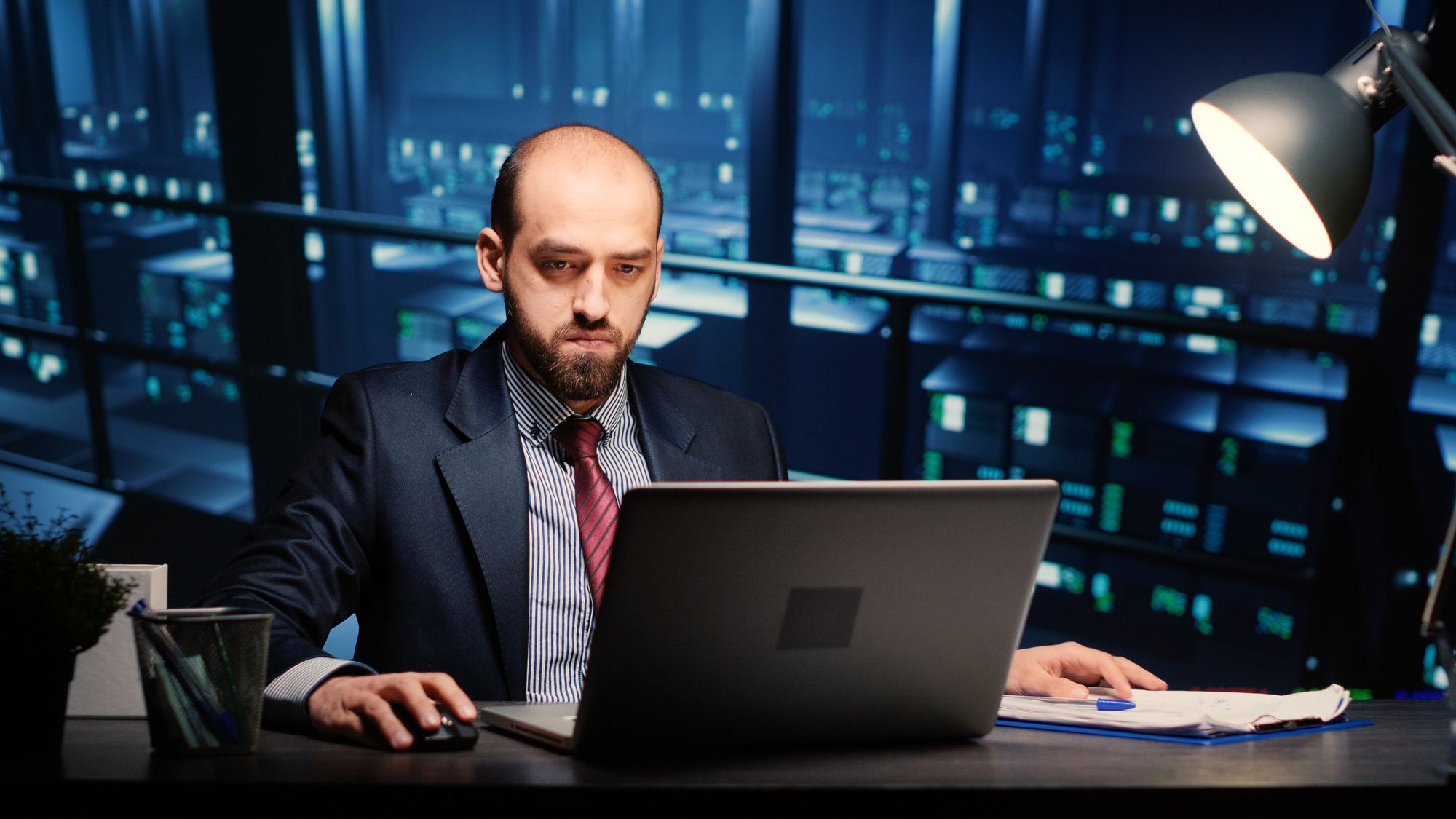
β
Data Engineers are responsible for designing and building data infrastructures that serve as the foundation for storing and managing vast datasets.
β
They create scalable and efficient architectures that can handle the ever-increasing volume of data generated by organizations.
β
The goal of data infrastructure is to ensure that data is organized, easily accessible, and can be processed efficiently for various analytical purposes.
β
2. Data Pipelines
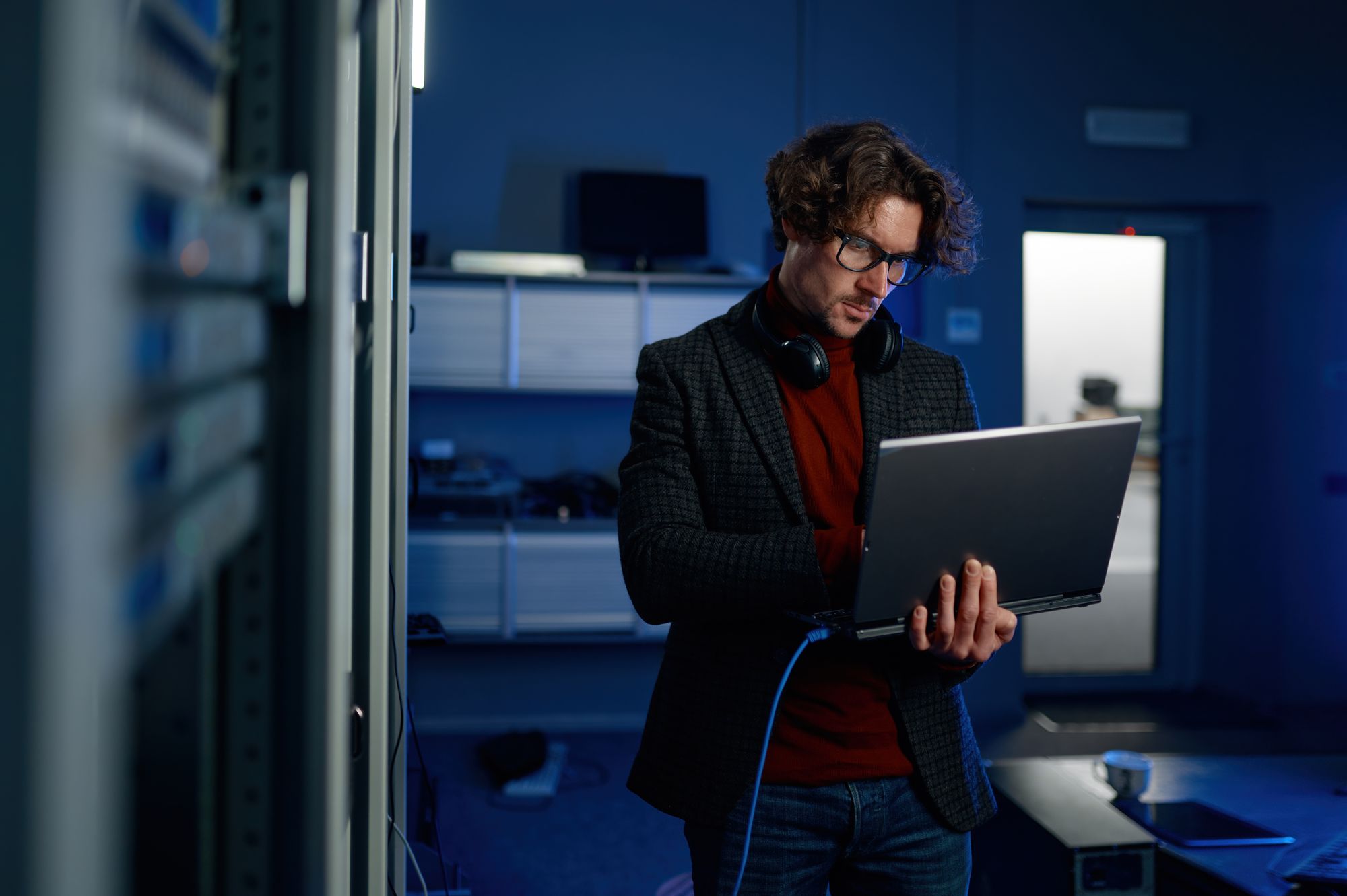
β
Data Engineers design, develop, and maintain data pipelines, which are critical for the smooth flow of data from its source to its destination.
β
These pipelines are essentially a series of data processing steps that transform and move data between different systems and components.
β
Data pipelines have a central role in data integration, as they facilitate the consolidation and synchronization of data from diverse sources, including databases, applications, and external APIs. This can occur in either real-time or batch-processing scenarios.
β
Through the orchestration of these pipelines, Data Engineers enhance the efficiency of data movement, minimize data delay, and improve data precision.
β
3. Data Warehousing
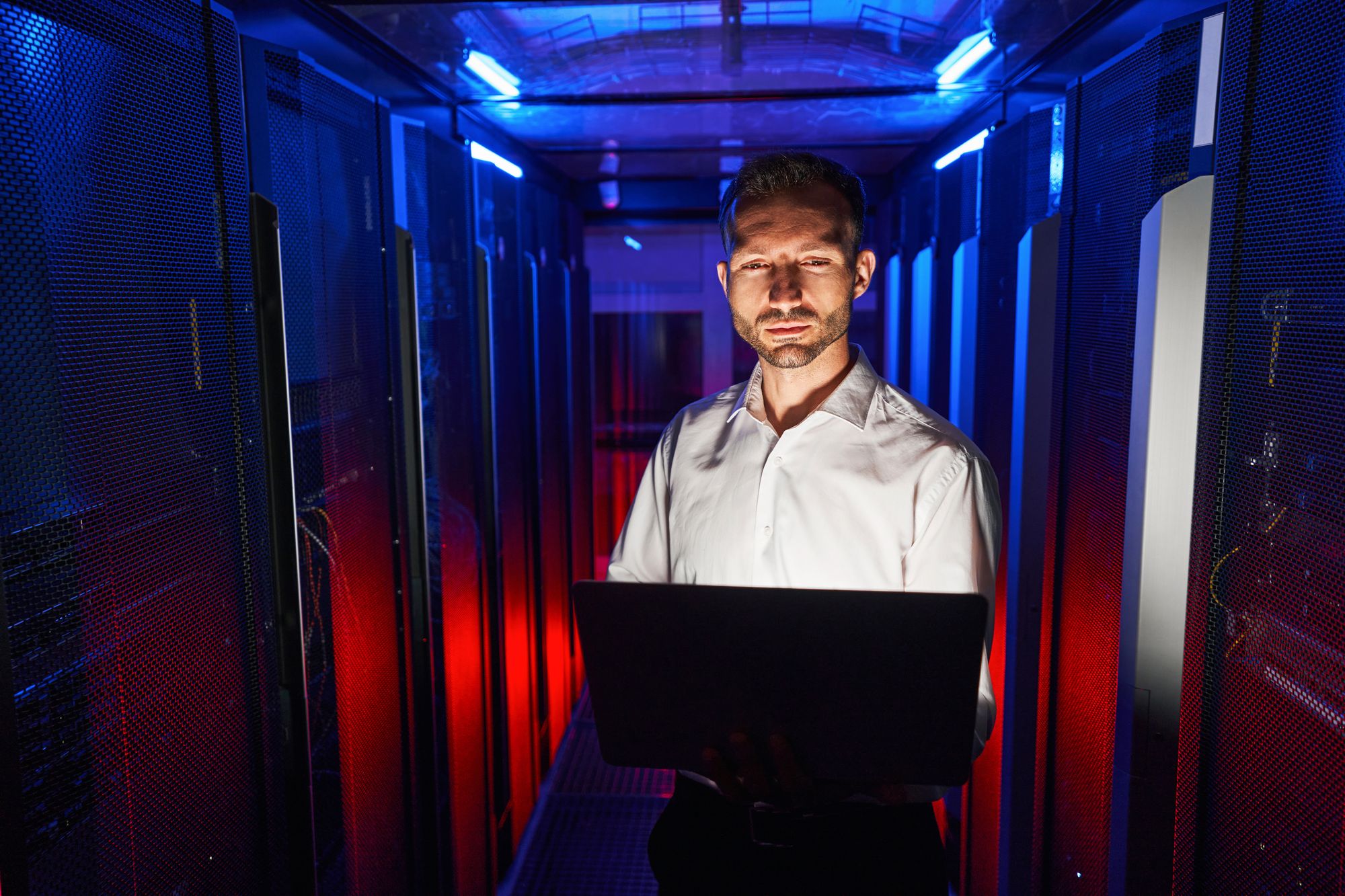
β
Data Engineers are involved in the creation and management of data warehouses, which are specialized databases designed to store and organize large volumes of structured and unstructured data.
β
Data warehouses provide a centralized repository that supports analytical queries and reporting.
β
They act as a foundational resource for Data Scientists and analysts, enabling them to efficiently access and analyze data for insights and decision-making.
β
4. ETL Processes
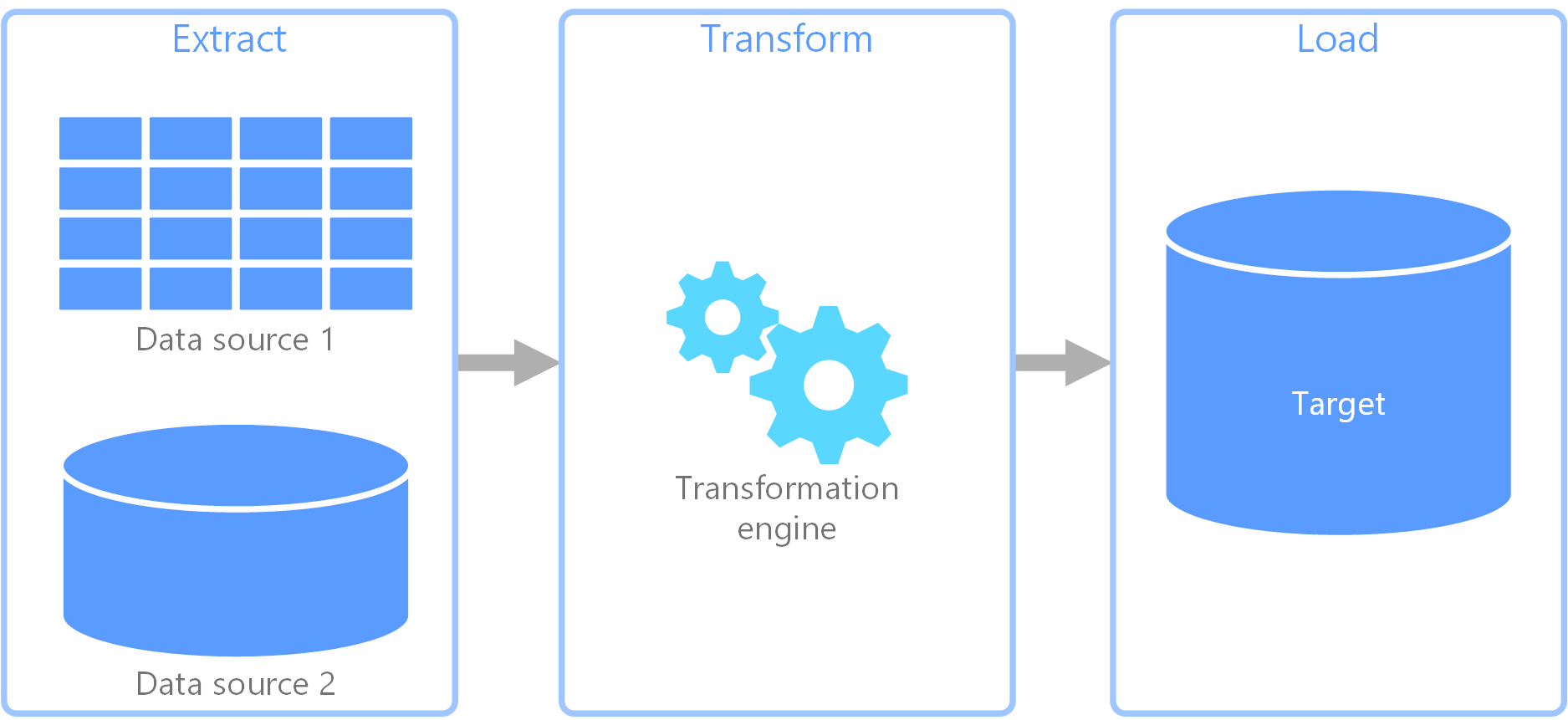
β
Data Engineers are experts in implementing Extract, Transform, and Load (ETL) processes, a vital component of data integration.
β
ETL is a three-step process that involves extracting data from various sources, transforming it into a standardized format, and loading it into a target system or database for analysis.
β
5. Data Quality Assurance

β
Data Engineers place a strong emphasis on data quality assurance to ensure that data is accurate, reliable, and fits its intended purpose.
β
They employ various data validation and verification techniques to detect and rectify inconsistencies or errors in the data.
β
Data quality assurance involves performing data profiling, data cleansing, and data validation processes to identify and rectify issues related to missing values, duplicates, outliers, and other anomalies.
β
6. Big Data Technologies

β
Data Engineers work with big data technologies such as Hadoop, Apache Spark, and other distributed computing frameworks to handle and process large-scale data efficiently.
β
In the era of massive data generation, Big Data Technologies have become essential tools for Data Engineers to handle and process large-scale datasets efficiently.
β
With the aid of these sophisticated Big Data Technologies, Data Engineers are empowered to dissect intricate data processing duties into more compact, manageable segments. These segments can then be executed concurrently across numerous nodes.
β
This approach boosts data processing speed, reduces data latency, and allows organizations to analyze vast datasets promptly.
β
7. Cloud Platforms
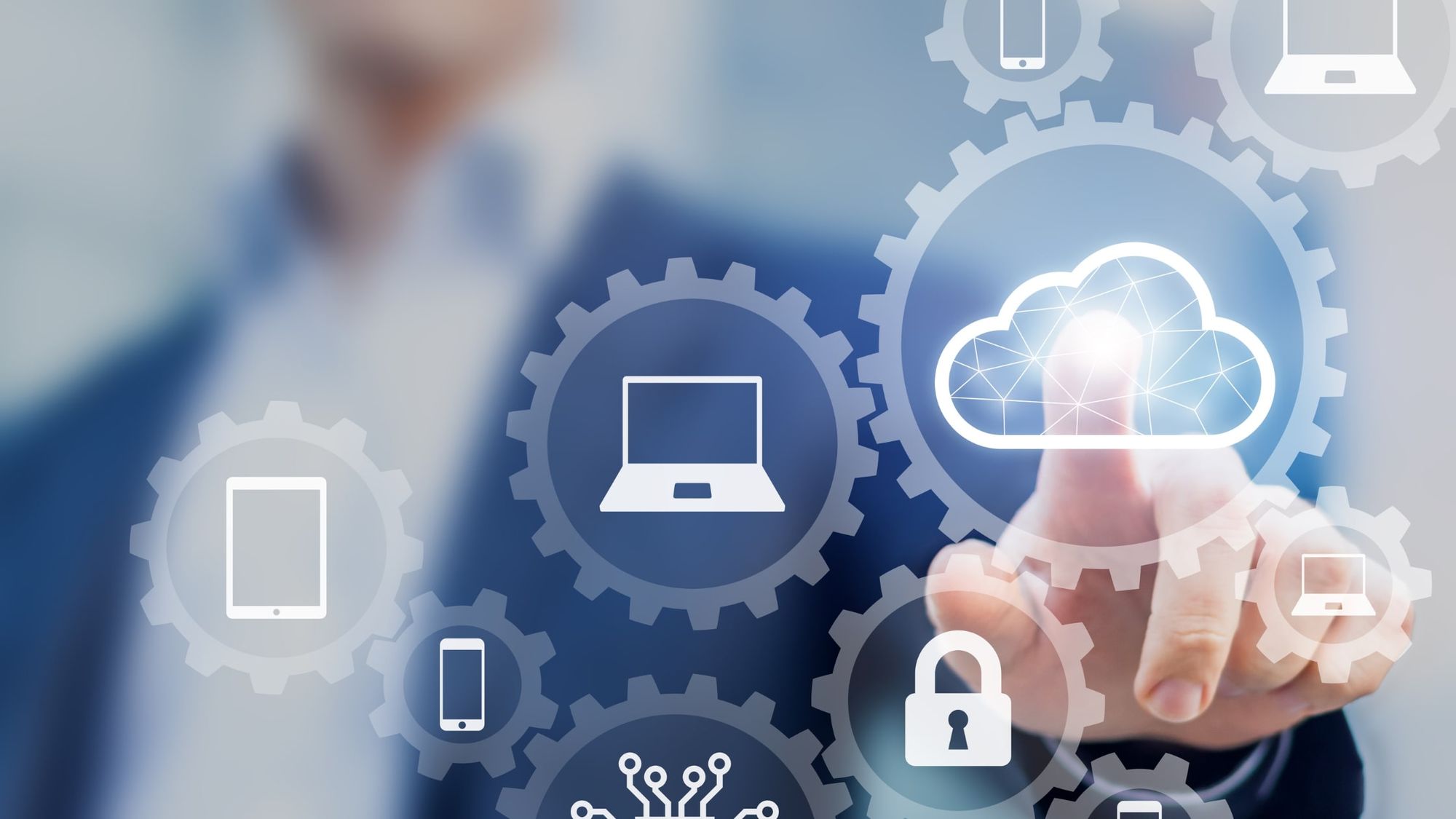
β
Cloud computing has revolutionized the way organizations manage and process data. Many Data Engineers turn to cloud-based solutions such as Amazon Web Services (AWS), Microsoft Azure, or Google Cloud Platform (GCP) to design scalable and cost-effective data solutions.
β
Cloud platforms offer a wide range of services and resources that allow Data Engineers to quickly provision, scale, and manage data infrastructure without the need for extensive physical hardware.
β
Cloud platforms provide Data Engineers with flexible and elastic computing resources, allowing them to handle fluctuations in data demand seamlessly.
β
8. Data Security and Compliance
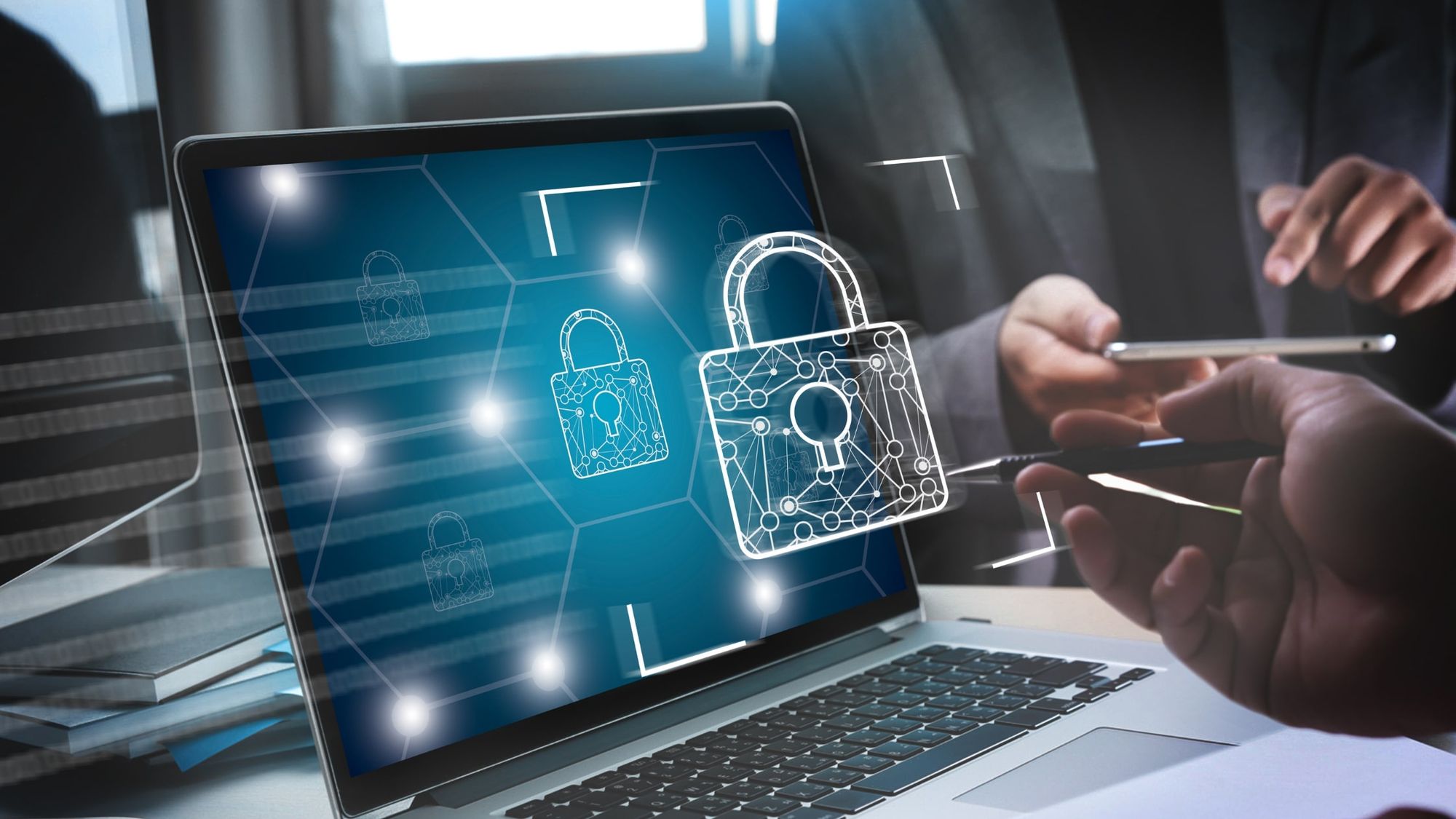
β
Data security and compliance are paramount concerns for Data Engineers when dealing with sensitive and confidential information.
β
As custodians of data infrastructure, Data Engineers implement robust data security measures to safeguard data from unauthorized access, data breaches, and cyber threats.
β
They utilize encryption techniques, access controls, and identity management solutions to protect data at rest and in transit.
β
Moreover, Data Engineers play a crucial role in ensuring compliance with data privacy regulations and industry standards. They implement data governance practices and audit trails to track data access and usage, ensuring data integrity and accountability.
β
9. Automation and Optimization

β
Data Engineers are skilled in automating data tasks and optimizing data processes to streamline data workflows and improve overall efficiency.
β
Through the automation of recurrent data-related responsibilities, like data extraction, transformation, and loading (ETL) procedures, Data Engineers diminish the necessity for manual involvement. This, in turn, mitigates the potential for human mistakes and conserves precious time and resources.
β
Automation also facilitates data updates on a regular schedule, ensuring that data remains up-to-date and relevant for analysis.
β
10. Collaboration

β
Data Engineers work collaboratively with various cross-functional teams to understand their data needs and design data systems that support their requirements.
β
Close collaboration with Data Scientists, analysts, and IT professionals is essential for Data Engineers to comprehend the specific use cases and analytical goals of each team.
β
Why Data Engineering is Important
β
Data Engineering plays a pivotal role in the success of organizations, serving as the backbone that supports the entire data ecosystem.
β
Data Engineers facilitate smooth data flow, enabling Data Scientists to focus on analysis rather than data management.
β
Here are several reasons why Data Engineering is crucial:
β
1. Seamless Data Accessibility
β
Data Engineering ensures that data is readily accessible to all relevant stakeholders within the organization.
β
Data Engineers create strong data architectures and pipelines, facilitating swift data access for Data Scientists, analysts, and team members.
β
This efficiency expedites data discovery, saving time and effort previously used for data searching and collection.
β
2. Efficient Data Processing
β
Data Engineering optimizes data processing and storage, allowing organizations to handle vast volumes of data efficiently.
β
With well-structured data pipelines, data can be ingested, transformed, and loaded into storage systems or data warehouses in real-time or batch processing.
β
This efficiency enables timely data updates and ensures that data is always up-to-date and relevant for analytical purposes.
β
3. Data Quality and Consistency
β
Data Engineers implement data quality checks and data validation processes to ensure the accuracy and reliability of the data.
β
By enforcing data quality standards, they prevent the introduction of erroneous or incomplete data into the system.
β
This attention to data quality ensures that insights and decisions derived from the data are based on reliable and trustworthy information.
β
4. Scalability and Flexibility
β
As organizations' data needs grow, Data Engineering ensures that the data infrastructure can scale accordingly.
β
Data Engineers employ scalable technologies and cloud-based solutions that can handle increasing data volumes and adapt to changing business requirements.
β
This scalability ensures that data operations can grow seamlessly alongside the organization's expansion.
β
5. Automation and Streamlining
β
Data Engineering involves automating repetitive data tasks and optimizing data processes, leading to increased efficiency and reduced manual intervention.
β
Automated data pipelines allow for scheduled data updates, reducing the need for manual data extraction and processing.
β
This streamlining of data operations frees up resources and allows Data Scientists to focus on their core expertiseβdata analysis and insights extraction.
β
6. Data Governance and Compliance
β
Data Engineering plays a vital role in ensuring data governance and compliance with data privacy regulations and industry standards.
β
With the help of implementing data security measures and access controls, Data Engineers protect sensitive data from unauthorized access and data breaches.
β
This adherence to data governance principles builds trust among users and ensures that data is handled responsibly and ethically.
β
7. Data-Driven Decision-Making
β
Data Engineering builds a robust data foundation, furnishing dependable, top-tier data to Data Scientists and stakeholders.
β
This empowers organizations to make well-informed decisions rooted in precise insights, driving business strategies, process optimization, and innovation.
β
What do Data Scientists Do?
β
Data Scientists are data wizards who possess a unique blend of analytical skills, domain expertise, and advanced technical knowledge.
β
Their primary mission is to dig deep into the vast ocean of data and uncover valuable patterns, trends, and insights that may remain hidden to the untrained eye.
β
Key responsibilities of Data Scientists include:
β
1. Data Analysis

β
Data Scientists are skilled at examining and exploring complex datasets to gain a deep understanding of the data.
β
They employ various statistical techniques, data visualization tools, and exploratory data analysis to identify patterns, correlations, and anomalies.
β
This initial data analysis helps them form hypotheses and guide their subsequent investigations.
β
2. Machine Learning and Predictive Modeling
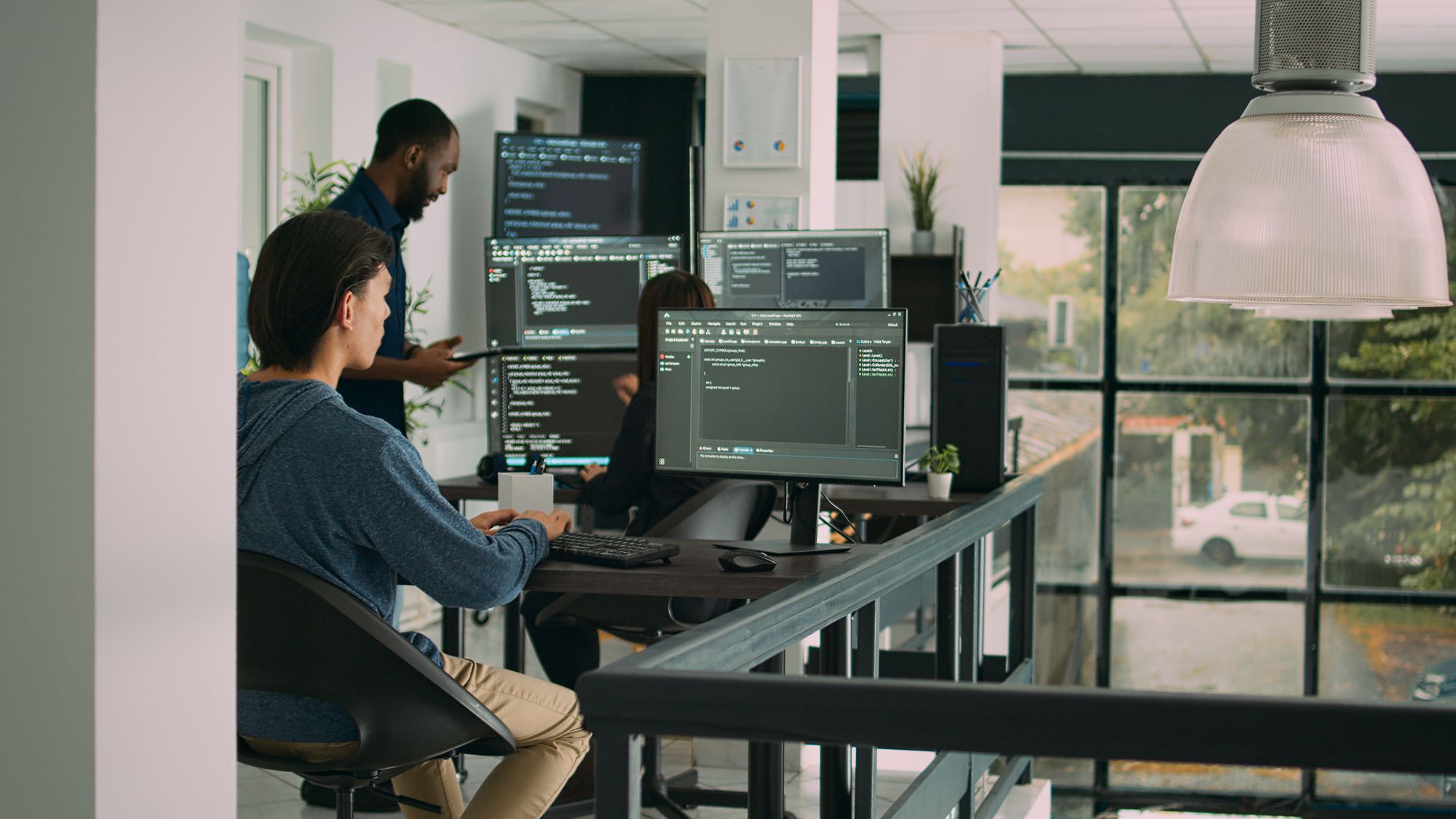
β
One of the key strengths of Data Scientists lies in their ability to leverage machine learning algorithms and predictive modeling techniques.
β
They build and train sophisticated models that can make predictions and provide valuable insights into future trends and outcomes.
β
These predictive models are essential for tasks such as demand forecasting, customer behavior analysis, and risk assessment.
β
3. Statistical Analysis

β
Data Scientists are well-versed in statistical methods and hypothesis testing, enabling them to validate their findings and draw meaningful conclusions from data.
β
By conducting statistical analyses, they can determine the significance of relationships between variables and make data-backed decisions.
β
4. Data Visualization

β
Data visualization is a powerful tool in a Data Scientist's arsenal.
β
They use charts, graphs, and interactive visualizations to present complex data in an easily understandable and actionable format.
β
Data visualization aids in effectively communicating insights to stakeholders, making information accessible and compelling.
β
5. Decision Support
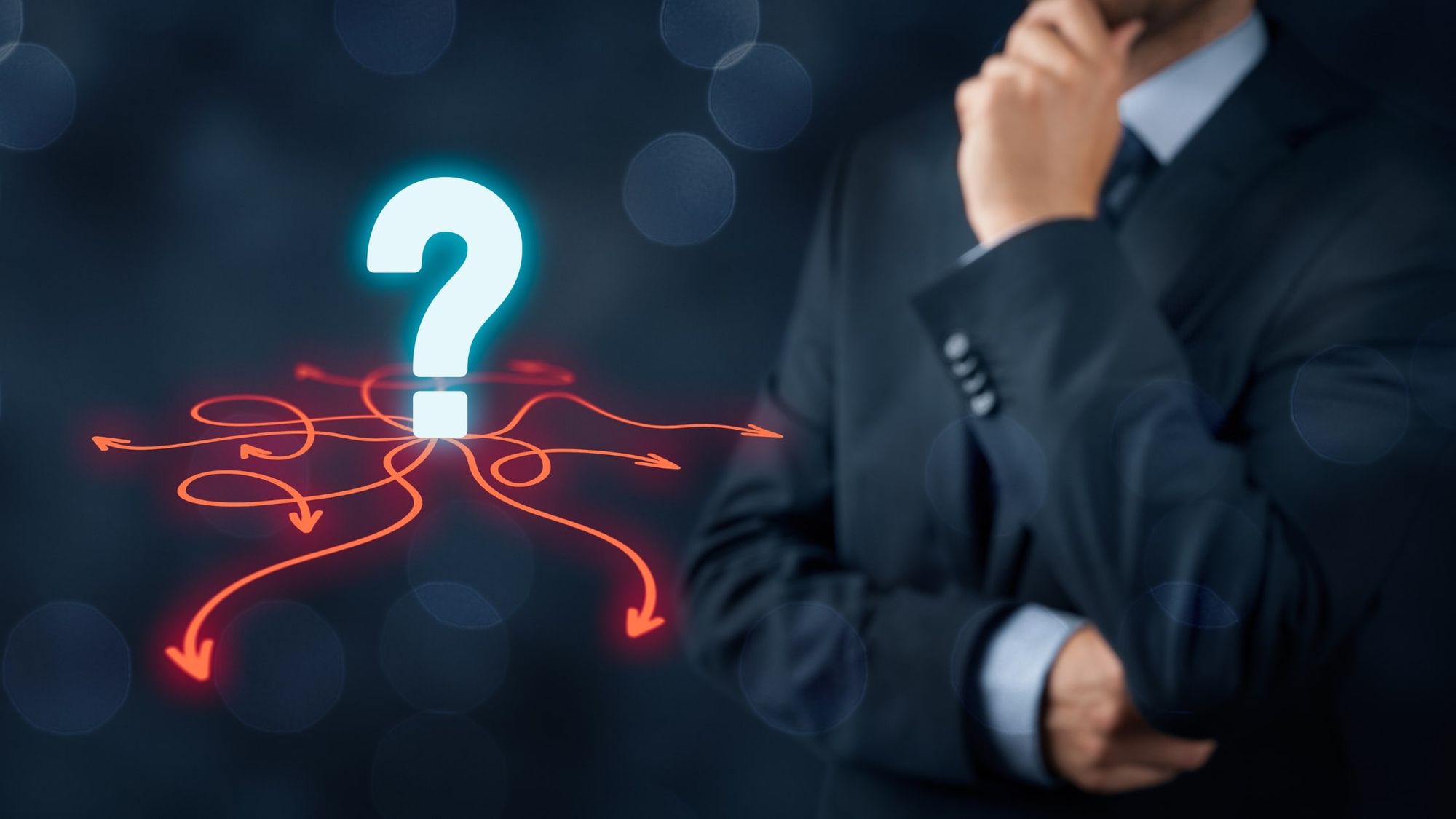
β
Data Scientists play a critical role in providing decision support to various business units and organizational leadership.
β
They generate actionable insights from data analysis, allowing decision-makers to make informed choices and devise effective strategies.
β
Data-driven decision-making helps businesses optimize processes, improve efficiency, and achieve their objectives more efficiently.
β
6. Model Optimization and Interpretation
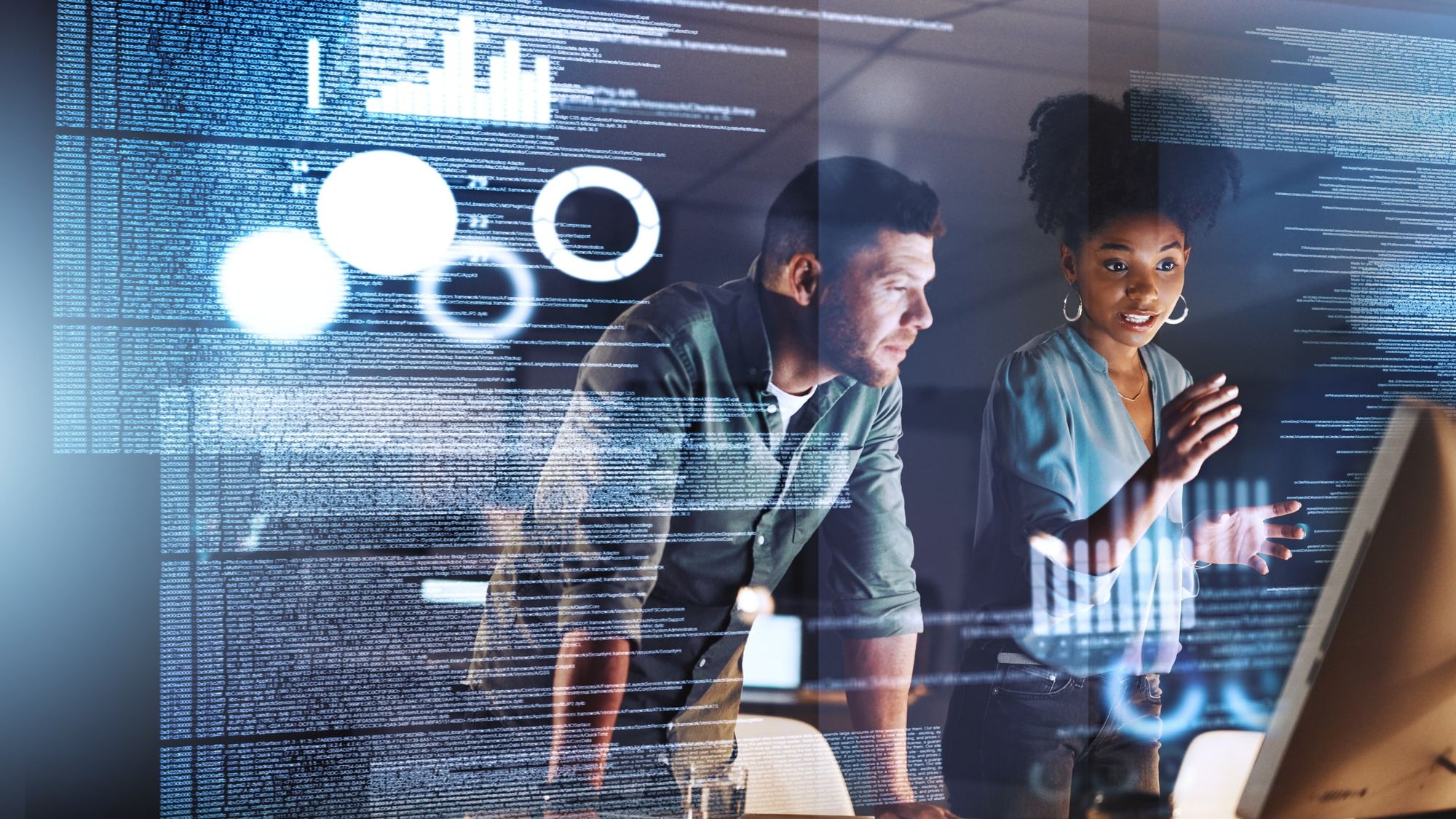
β
Data Scientists continuously work on refining and optimizing their models to improve accuracy and performance.
β
This iterative process involves tweaking model parameters, experimenting with different algorithms, and fine-tuning model architectures.
β
Furthermore, they interpret model outputs and identify factors that contribute most significantly to the predictions, providing valuable insights into the underlying mechanisms.
β
7. Real-world Applications

β
Data Scientists have a diverse range of applications across various industries. They contribute to fields such as healthcare, finance, marketing, cybersecurity, and more.
β
For example, in healthcare, Data Scientists may analyze medical data to predict disease outcomes or optimize treatment plans. In marketing, they may analyze customer data to personalize marketing campaigns and improve customer experiences.
β
8. Continuous Learning
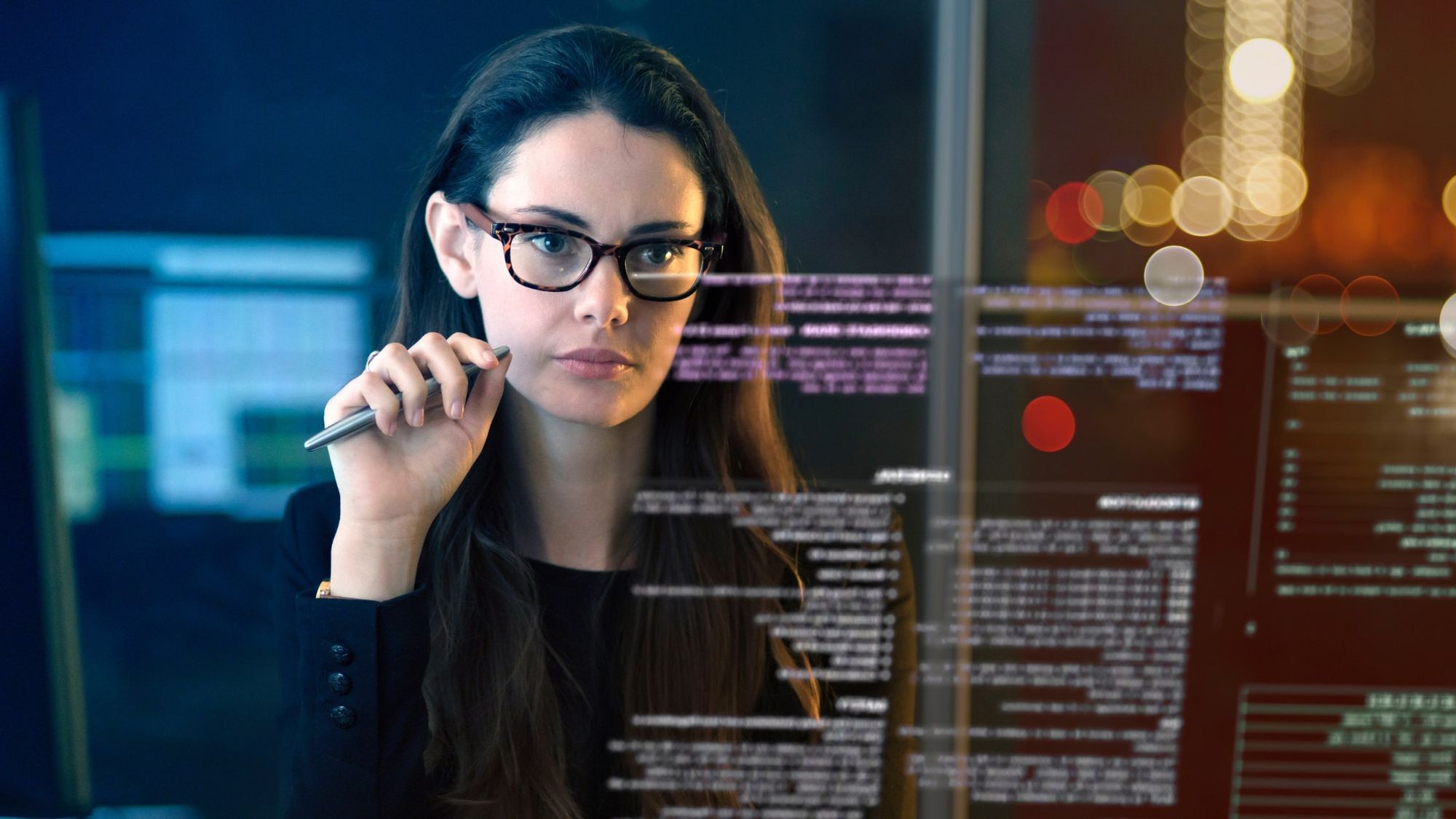
β
Data Science is a rapidly evolving field, with new techniques, tools, and technologies emerging regularly.
β
Data Scientists invest time in continuous learning and staying up-to-date with the latest trends to ensure they are equipped with cutting-edge skills and knowledge.
β
This commitment to learning allows them to adapt to evolving data challenges and remain at the forefront of data analysis.
β
Why Data Science is Important
β
Data Science empowers organizations to transform data into actionable knowledge.
β
By leveraging advanced analytical techniques, Data Scientists drive innovation, optimize processes, and unlock valuable insights, contributing to the organization's growth and success.
β
Here are some key aspects that highlight the significance of Data Science:
β
1. Extracting Insights from Data
β
Data Science enables organizations to gain valuable insights from their vast and diverse datasets.
β
Data Scientists use a combination of statistical analysis, data modeling, and machine learning algorithms to extract meaningful patterns, correlations, and trends from the data.
β
These insights provide a deeper understanding of customer behavior, market trends, operational inefficiencies, and other critical aspects that impact the organization's performance.
β
2. Driving Innovation
β
Data Science drives innovation by extracting insights from data. Data Scientists pinpoint chances for refining processes, developing products, and enhancing services within organizations.
β
They use predictive modeling to anticipate future trends and customer demands, leading to the development of innovative products and solutions that cater to evolving market needs.
β
3. Optimizing Decision-Making
β
Data Science plays a crucial role in supporting data-driven decision-making.
β
By providing evidence-based insights, Data Scientists enable organizations to make informed choices and strategic decisions.
β
Whether it's devising marketing strategies, resource allocation, or operational improvements, information-centric decision-making minimizes risks and enhances the likelihood of successful outcomes.
β
4. Personalizing Customer Experiences
β
Data Science enables organizations to deliver personalized and tailored customer experiences.
β
Data Scientists analyze customer data, encompassing past actions, preferences, and engagements, to categorize customers and devise focused marketing campaigns. Customized encounters enhance customer engagement, loyalty, satisfaction, and retention.
β
5. Identifying Business Opportunities and Risks
β
Data Science aids in identifying potential business opportunities and risks.
β
Organizations analyze market data and industry trends to reveal new markets, emerging trends, and growth prospects.
β
This analysis also identifies potential risks like operational inefficiencies, market shifts, and cybersecurity threats, enabling proactive mitigation.
β
6. Improving Operational Efficiency
β
Data Science helps optimize business processes and operational efficiency.
β
Through the examination of production, supply chain, and resource data, organizations spot bottlenecks and inefficiencies. Insights derived from data drive enhancements in processes, resource allocation, and cost efficiency, ultimately boosting operational effectiveness.
β
7. Enhancing Product and Service Quality
β
Data Science enables organizations to continuously monitor and improve the quality of their products and services.
β
Data Scientists use customer feedback, usage data, and performance metrics to pinpoint improvement opportunities. This iterative method in product and service development guarantees customer-centricity and sustains a competitive market advantage.
β
8. Predictive Analytics
β
Predictive analytics is a key component of Data Science that enables organizations to anticipate future trends and outcomes.
β
Data Scientists construct predictive models using historical data to anticipate customer behavior, demand trends, and market shifts, empowering proactive decisions that provide a competitive edge to organizations.
β
Comparing Data Engineers and Data Scientists
β
While both roles revolve around data, Data Engineering and Data Science serve distinct purposes.
β
Data Engineering focuses on building and maintaining the infrastructure that supports data processing and storage, ensuring data accessibility and reliability.
β
Data Science, on the other hand, revolves around analyzing data to extract insights, generate predictions, and support decision-making.
β
For a comprehensive review, here's a side-by-side comparison of a data engineer and data scientist:
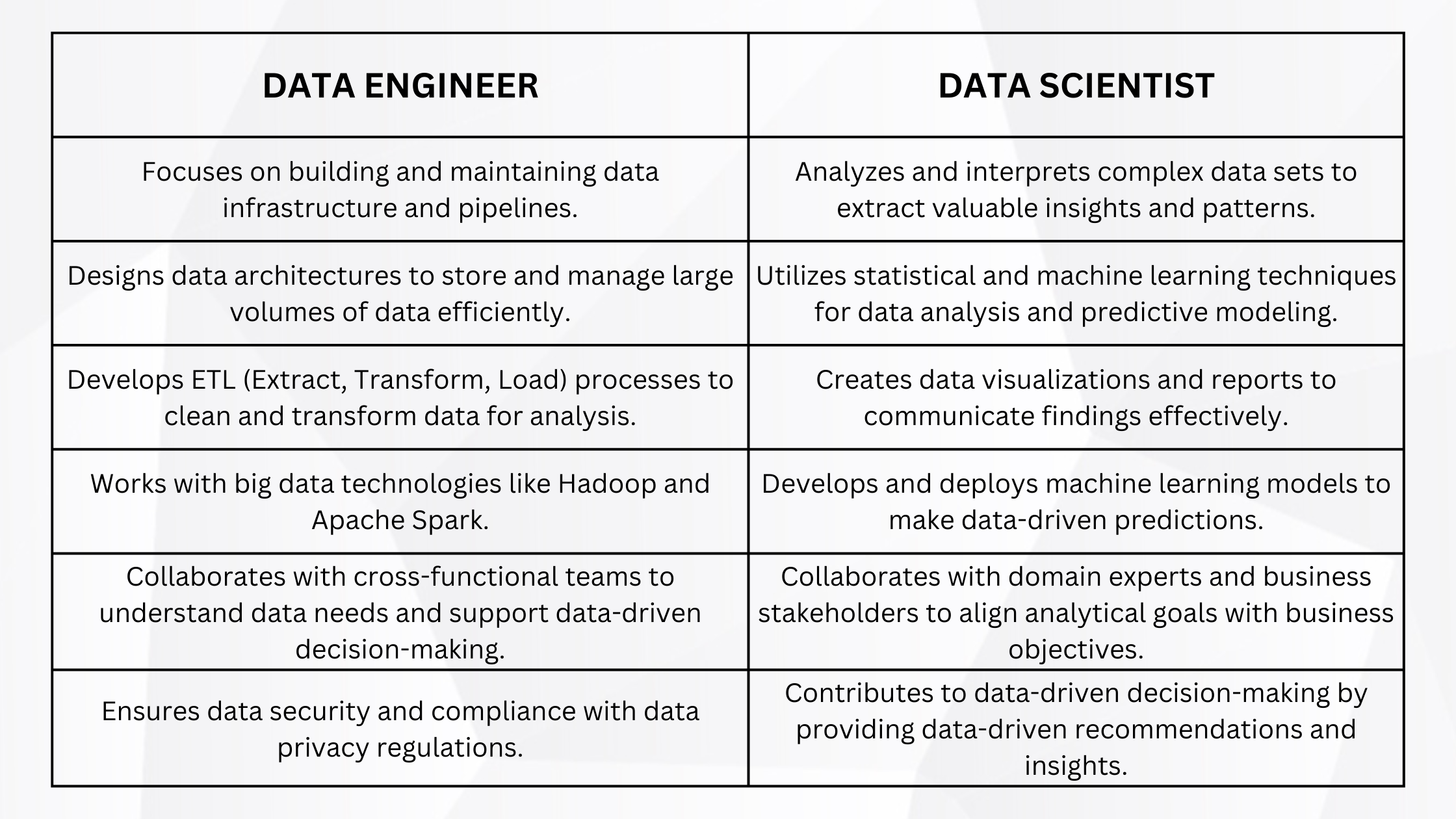
β
The Future Demand for Both Roles
β
The future demand for both Data Engineers and Data Scientists is poised for significant growth due to the ever-increasing importance of data in modern business operations and decision-making processes.
β
Several key factors contribute to the exponential rise in demand for these roles:
β
Data-Driven Decision-Making
β
Businesses across industries are increasingly recognizing the value of data-driven decision-making.
β
Data provides valuable insights into customer behavior, market trends, operational efficiency, and more.
β
As organizations strive to remain competitive and agile, the need for professionals who can effectively handle and analyze data becomes crucial.
β
Technological Advancements
β
Advancements in technology have led to the generation of vast amounts of data from diverse sources.
β
With the proliferation of the Internet of Things (IoT), social media, and connected devices, the volume, velocity, and variety of data have grown exponentially.
β
Data Engineers are instrumental in designing scalable and efficient data infrastructures to handle this explosion of data.
β
Artificial Intelligence and Machine Learning
β
The adoption of artificial intelligence (AI) and machine learning (ML) is on the rise across various industries.
β
Data Scientists play a pivotal role in leveraging these technologies to develop predictive models, automate processes, and uncover patterns in data.
β
The integration of AI and ML into business processes will further drive the demand for skilled Data Scientists.
β
Industry-Specific Applications
β
Different industries are increasingly leveraging data analytics for industry-specific applications.
β
For example, healthcare organizations use data to enhance patient outcomes, financial institutions use data to detect fraudulent activities, and e-commerce companies use data for personalized customer experiences.
β
Each of these applications requires specialized Data Engineers and Data Scientists with domain expertise.
β
Data Privacy and Security
β
With the increasing concern around data privacy and security, businesses need experts to ensure that data is handled responsibly and in compliance with regulations.
β
Data Engineers play a vital role in implementing data security measures, while Data Scientists must also be mindful of data privacy when handling sensitive information.
β
Adoption of Cloud Computing
β
The adoption of cloud computing has revolutionized data storage and processing. Many organizations are moving their data infrastructure to cloud platforms to take advantage of scalability, cost-effectiveness, and ease of management.
β
Data Engineers with expertise in cloud-based solutions will be in high demand to design and implement these systems.
β
Demand Across Industries
β
Data-driven decision-making and data analytics are not limited to any specific industry.
β
Every sector, including healthcare, finance, retail, manufacturing, and more, can benefit from harnessing the power of data.
β
Consequently, the demand for skilled Data Engineers and Data Scientists spans across various industries.
β
Conclusion
β
Data Engineers and Data Scientists are more than just job titles; they are the enablers of data-driven excellence, transforming data into actionable knowledge and empowering organizations to make informed decisions in an increasingly data-centric world.
β
Data Engineers form the backbone by designing and implementing robust data infrastructure and pipelines, ensuring data is efficiently processed and stored.
β
On the other hand, Data Scientists are the analytical minds who extract valuable insights from the data, using statistical analysis and machine learning techniques to drive innovation and inform decision-making.
β
The synergy between these roles is what makes the analytics-informed revolution possible. Data Engineers create the foundation on which Data Scientists can build their analyses, and Data Scientists depend on the quality and accessibility of data provided by Data Engineers.
β
Their collaboration allows businesses to build a solid infrastructure and unlock the full potential of their data. This enables them to gain a competitive edge, identify new opportunities, and address challenges proactively.
β
Ready to supercharge your data capabilities? Get in touch with Pro5 and equip your team with top data engineers and data scientists today!
β